Tentang KamiPedoman Media SiberKetentuan & Kebijakan PrivasiPanduan KomunitasPeringkat PenulisCara Menulis di kumparanInformasi Kerja SamaBantuanIklanKarir
2025 © PT Dynamo Media Network
Version 1.94.1
Konten dari Pengguna
Beyond GDP: Preventing Data Radicalism and Pseudoscience
28 April 2024 9:44 WIB
·
waktu baca 3 menitTulisan dari Leonardo tidak mewakili pandangan dari redaksi kumparan
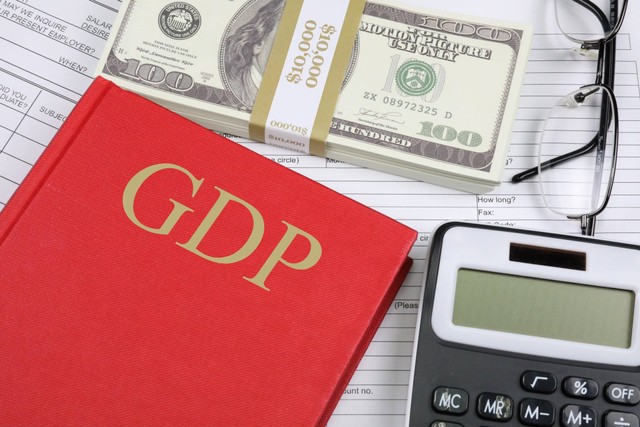
ADVERTISEMENT
Since first introduced by Simon Kuznets in 1934, Gross Domestic Product (GDP) has become one of the most popular indicators for measuring a country's economic success. However, it is not uncommon for GDP to be used as a sole indicator to predict success in whole contexts, such as happiness, health, and well-being. Indeed, this indicator has become the most popular in achieving the Sustainable Development Goals (SDGs) 2030 (Coscieme et al., 2020 ). Therefore, it is not surprising that many countries, through their leaders, are competing to pursue the highest GDP.
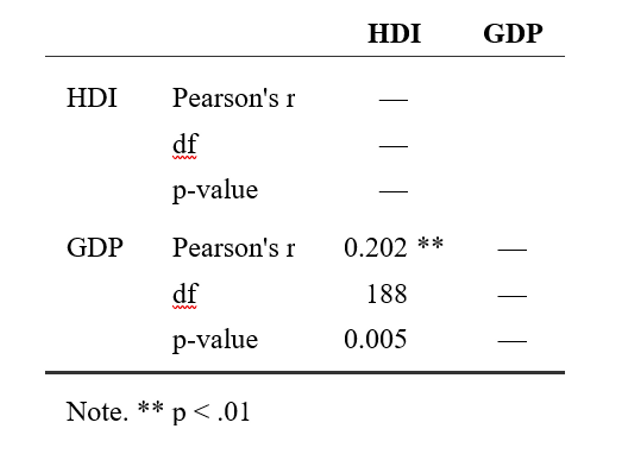
However, GDP cannot always predict various levels of non-economic well-being, such as the Human Development Index (HDI), as in Table 1. Data processing conducted by the author from 190 countries shows that the correlation index and effect size of GDP on HDI is relatively tiny, with each value of 0.202 and 0.043. It means that only 4% of HDI improvement can be predicted by GDP. Therefore, basing a country's success solely on economic indicators, especially GDP, is problematic and potentially leads to pseudoscience. So, what are holistic and comprehensive methods to achieve the SDGs 2030?
ADVERTISEMENT
Unlike scientific methods, data-driven approaches do not always have to be based on empirical facts (Mahayana, 2022). Thus, it makes data-driven approaches the preferred method for forming conclusions nowadays, generally in the era of big data. Some extremists believe the scientific approach has ended and transitioned into the data era. Furthermore, there is no longer a need for theories or models to explain a phenomenon and its causality. Correlative conclusions are sufficient as a basis for decision-making. "Let the data explain itself" roughly describes the form of data radicalism.
However, data radicalism contradicts Laden's Theory, which states that data is inherently biased and cannot explain itself (Bogen, 2009 ). Therefore, a theoretical framework and scientific method are needed to construct it. It explains why there can be disparities in data demonstrating the same phenomenon. In this context, the existence and wisdom in selecting theoretical foundations become significant in obtaining data that most accurately represents reality.
ADVERTISEMENT
Disasters in the economic and global markets due to data radicalism have occurred several times worldwide. In 2011, Google released a program to predict flu trends in the United States called Google Flu Trends (GFT) (Lazer et al., 2014 ). This prediction was based on internet users' number of clicks on flu-related information. However, there was an overprediction that led to the overproduction of flu medication, causing many pharmaceutical companies to suffer losses because they could not meet the demand of the fictitious market. Eventually, GFT was shut down because users' feedback could not correct its inaccuracies.
Furthermore, in Indonesia, there was a case of trading bots involving "Crazy Rich Medan," Indra Kenz . He promoted a trading application that claimed to predict the stock market, thus providing a shortcut to wealth. However, the artificial intelligence-based application only relied on predictive correlation analysis, which contradicts the Efficient-Market Hypothesis: the stock market fundamentally cannot be predicted. It resulted in public losses of up to $5.3 million and criminal punishment for "Crazy Rich."
ADVERTISEMENT
The various apocalyptic phenomena mentioned above indicate that the scientific method, with its falsification, is greatly needed so that data science does not become pseudoscience. Moreover, reality must be more comprehensively approached inductively and positivistically. Indeed, this has been applied in natural science, especially modern physics, in Einstein's Theory of Relativity, which embraces imaginative aspects: gravity is the curvature of space and time (French, 2020 ).
In the context of social sciences, such as economics, embracing diverse positivist indexes can be a solution to prevent data radicalism and its various negative impacts. GDP should not necessarily be the sole indicator of a country's success, especially regarding non-economic aspects affecting public welfare. Therefore, the global community, from research institutions to financial institutions to national leaders, can adapt alternative indexes to indicators of a country's success, such as HDI, Gini index, Multidimensional Poverty Index (MPI), etc.
ADVERTISEMENT
Moreover, accommodating non-positivist scientific approaches, such as case studies, ethnography, and phenomenology, can enrich the information that quantitative research cannot obtain. One advantage of qualitative research is its ability to identify unintended consequences of a policy, including economic (Haven & Grootel, 2019 ). It aligns with the aspiration for falsification in the scientific method to patch defects and improve a previously established conclusion.
Thus, instead of becoming data extremists, embracing epistemological diversity in reaching conclusions and policy foundations is the best way to achieve the diverse goals of the SDGs. Because life is not only composed of economic aspects, let alone GDP, life is holistic rather than purely positivistic.